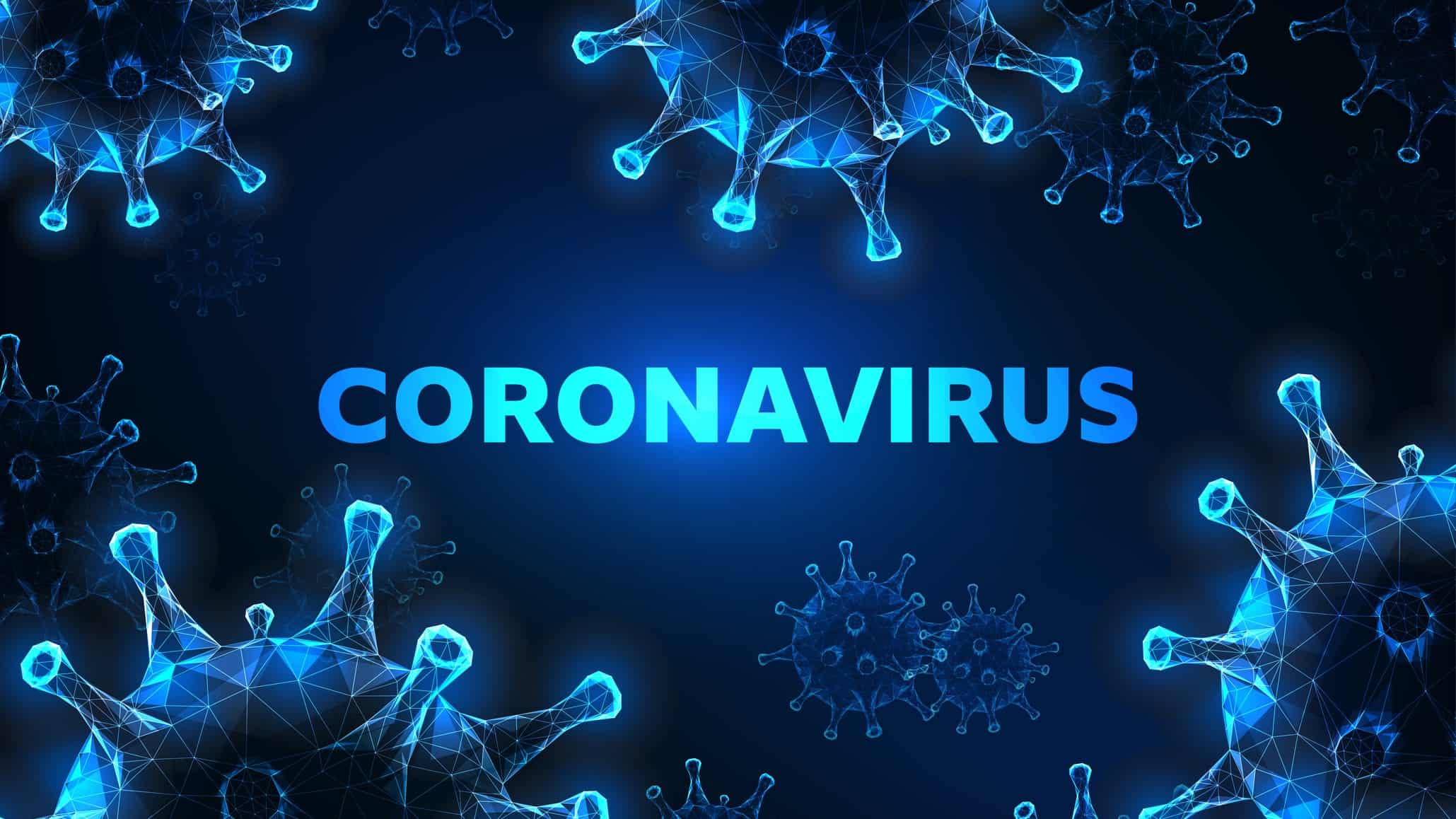
What is happening with all the data around the COVID-19?
Why is so much of the information we hear inaccurate? Is the data wrong? Are the data scientists wrong? What is happening?
With our 24-hour news cycle, we are bombarded by new findings regarding the Coronavirus. But just as hastily as the information is reported, it is swiftly deemed incorrect. What is the cause of this inaccuracy? Are we experiencing some fundamental flaw with big data?
It’s not that simple. In a panic, people are overreacting and putting information out there without fully comprehending it. This virus is novel. It was thrust upon us suddenly and has forced everyone indoors.
There hasn’t been a pandemic of this scale since the Spanish flu in 1918. We are in a far different, interconnected world, and the socio-economic effects of this pandemic have yet to be fully realized. For all intents and purposes, this is unfamiliar territory when it comes to the understanding of the data associated with it. The idiom, only time will tell, seems fitting at this time.
Some key factors to point out to gain a better understanding of big data regarding COVID-19:
Data from China – The initial models were built around data from China, and unfortunately, these were invariably softened or misrepresented numbers. As such, the preliminary counts were less alarming than what we’ve seen play out in our nation and across the world stage. In no way is this a statement of race or an indictment of China, but just an unfortunate by-product of our cultural and political differences.
Time is a crucial factor – It’s vital to understand that when it comes to the analysis and interpretation of big data, there is a required element; which is time. Time is a crucial factor in any data modeling. Since this virus has only been around for a few months, the case studies, including what exact physical circumstances make someone more vulnerable, are yet to be fully realized.
A Constellation of models – To better understand the larger picture of data, scientists study small segments. For example, they are analyzing data from a small town or community impacted and building models to understand better the data associated with the virus. From there, these short segments are combined to build a constellation of models with the end goal of finding the cornerstone for which to interpret the results.
The Hydroxychloroquine effect – You can look no further than the events around hydroxychloroquine to grasp how data analysis plays out. The initial claim that hydroxychloroquine was a cure was swiftly proven as time and cases were studied. It has since been debunked as not unable to cure the virus, but potentially being more harmful.
The Virus X-Factor – There is the additional x-factor that this is a virus, which is a living thing and, therefore, not a static data point with a high potential for mutation making the time and case-studies more challenging.
We still have quite a journey ahead of us, and errors in data analysis can turn into substantial significant factors. There is an exponential curve at play that is making things very sensitive. Early results were guessing; the passing of time and gaining of knowledge and cases will ultimately result in inaccuracy in predictions. As time passes and we collect more data, the result will be a better understanding, analysis, and eventually, a cure to this pandemic.
The essential piece of data to recognize is the authentic and tragic element of this pandemic that all of these points of data are actual human lives: friends, relatives, loved-ones whose lives are forever changed or lost by this catastrophe.
The way we live our lives, conduct our business, educate our children is forever altered. We are forever changed by the events of the last few months. But as human beings, we persevere and adapt. We will emerge from this more robust, resilient, and determined.
The data we gather about our lives is no longer a scary, “Big Brother” thing, but will come to serve us, and aid is in rebuilding our lives and plotting our new course.
For now, we will use the information to stop the spread, modify our interactions, and ultimately develop a cure.
Contributor to this article:
Jay Swartz – Executive Leader and Data Scientist. Jay enables companies to leverage machine learning (ML) and data science (DS) by building ML solutions as well as helping them to establish and extend skilled teams. He is the Chief Scientist at Likely.ai and advises companies as a Guidepoint Advisor specializing in AI/ML/DS.
From predictive modeling and complicated algorithms to deep learning and artificial intelligence, Likely.AI is a new-age lead generation tool that is changing lead quality expectations by identifying consumers’ likely real estate decisions before their decisions have been made. To learn more, you can contact Likely.AI here, or Here to Learn More About Our COVID19 Impacted Properties Dataset